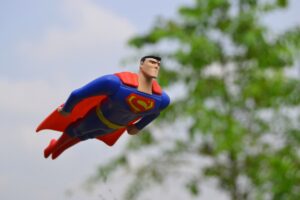
The Age of the Super-Recruiter
An Interview with Sanjoe Jose, CEO and Cofounder of Talview The challenges in hiring span from finding the right talent to finding the technology to
An Interview with Sanjoe Jose, CEO and Cofounder of Talview The challenges in hiring span from finding the right talent to finding the technology to
AI is projected to catapult from a $643.7 million market today to $36.8 billion by 2025. Bersin by Deloitte calls it one of the ten
It’s one thing to see AI coming our way in HR. It’s another thing to know the best ways to harness it to improve sourcing
HR and recruiters don’t tend to take things at face value. For good reason: we’re called on to rely on our educated judgments. We’re in
Advancements in machine learning are beginning to change the way organizations approach recruiting. Here’s what you need to know.
Companies approach recruiting in different ways. Facebook’s method, for example, involves acquisitions for human capital and a six-week onboarding boot camp. Of course, that’s not the
Interest in artificial intelligence (AI) recruiting technology has exploded recently. From finance to sales departments, business leaders are asking how they can leverage AI technology
I love big data. I love it for many reasons, but, as I’ve said before, one of the main reasons is the way it’s “raised the